AI and Third-Party Risk in EU Financial Sector
During a recent third-party risk evaluation, a client was surprised when asked about AI components in an ICT solution. The reason? Under DORA, AI suppliers must be assessed as part of the risk management framework. However, understanding what qualifies as AI under the AI Act remains a challenge for many.
Want to know how to stay compliant?
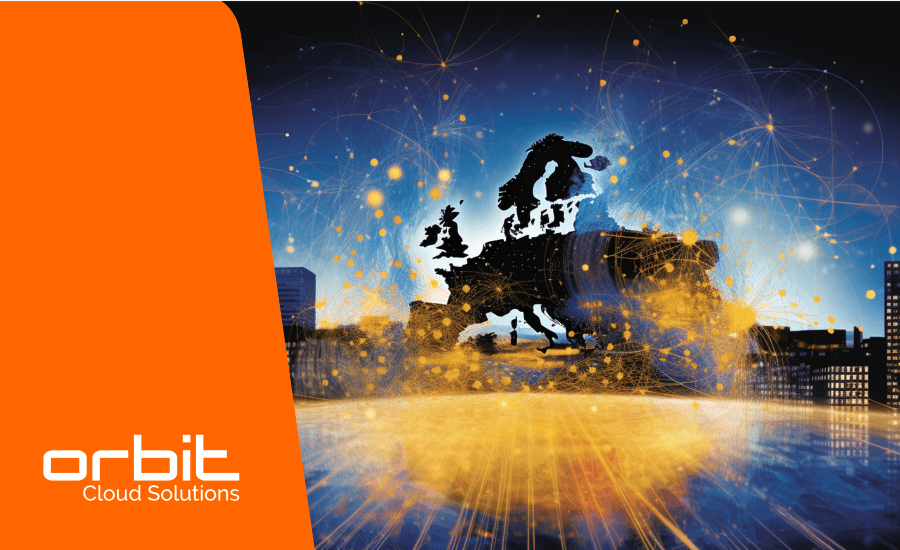
Double revelation: AI systems and third-party risk management in the EU financial sector
Third-party risk assessment involves evaluating the risks a potential business collaboration may pose to a company. This procedure is mandatory for all financial entities under DORA.
Financial sector is a traditionally highly risk-averse and heavily regulated industry. Consequently, this sector already has stringent cybersecurity regulations in place, while the remaining essential and important entities in other business sectors are still waiting to learn how requirements of NIS2 related to supply chain security will turn out for them in the national legislations.
Here is an example of the tough process around some of these requirements:
During a recent client meeting related to third-party-risk management, we went through a battery of questions related to an ICT solution to be purchased (an assessment mandated by DORA). The client experienced two key revelations that I believe are worth sharing.
First, they wondered why we inquired whether the IT solution involved AI, Machine Learning, or Large Language Model components. Second, they weren’t sure how to determine what is even considered AI and what not.
Now, we asked about AI/ML/LLM components for two reasons. Many applications use third-party supplied AI components. If this is the case, the AI component supplier must be assessed as a third party under the third-party risk management framework. Next, if AI is involved, further inquiries might be necessary to ensure compliance with the AI Act.
Secondly, our client was well informed about the problem of the definition of AI system as presented in the AI Act, which demonstrates that being informed may not be conductive to (or may even hinder) understanding.
Article 3 (1) of the AI Act defines AI system as a
“machine-based system that is designed to operate with varying levels of autonomy and that may exhibit adaptiveness after deployment, and that, for explicit or implicit objectives, infers, from the input it receives, how to generate outputs such as predictions, content, recommendations, or decisions that can influence physical or virtual environments.”
To grasp the regulatory concept, one must analyse the Draft Commission Guidelines on the definition of an artificial intelligence system, released in early February 2025. Although not formally adopted, these guidelines offer insight into the EC’s train of thought.
The EC’s notion of AI system is extensive and although the guideline lists several defining conditions, I would highlight only two of them as essential when it comes to helping understand the regulatory concept: autonomy, and “inferencing how to generate outputs”.
Both the AIA and the guidelines make it clear that for a computational solution to be considered “AI system”, it is not necessary that it is fully autonomous. The guideline states even a system that, “based on input provided by a human, to produce an output on its own such as a recommendation” fulfills the AIA condition to be “designed to operate with varying levels of autonomy”. For short, anything that doesn’t need to be fully operated by hand in each step of the process, fulfils this condition, and the level of its autonomy does not matter.
With respect to the somewhat clumsy formulation regarding inferencing, the guidelines state this means both “an ability of a system to derive outputs from given inputs, and thus infer the result.”, and also “the building phase, whereby a system derives outputs through AI techniques enabling inferencing.”
There are many solutions that would fulfil such a definition. One would even hypothesize this can include applications such as Microsoft excel. To prevent similar misconceptions, the guideline literally excludes “basic data processing”. Conversely, systems utilizing techniques such as deep-learning, reinforcement learning, machine learning but also ‘logic- and knowledge-based approaches’ are named as constitutive for an AI system.
These questions reveal a governance void. With robust AI governance, IT and security leaders won’t need to answer all these questions - designated business owners will. And with clear oversight of AI systems across the company. Nevertheless, since AI governance is still emerging, this insight will hopefully add value.